AI in Energy Management
- Julian Knight
- Oct 14, 2023
- 3 min read
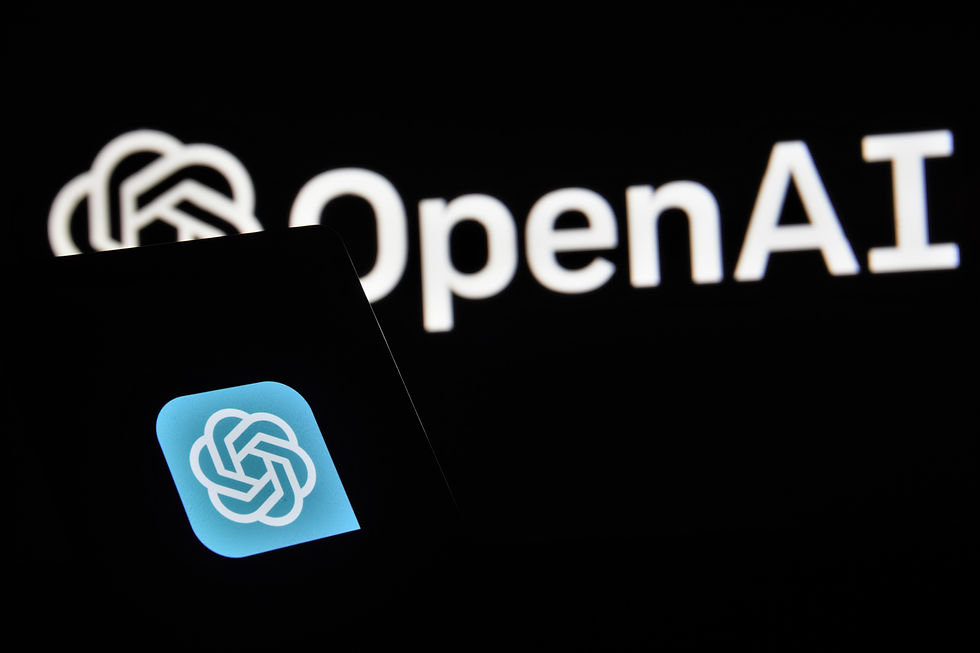
Written by: Julian Knight
Edited by: Ada Collins
Since the emergence of ChatGPT as an open source language processing tool in the late fall of 2022, artificial intelligence (AI) has become readily available to the public. AI tools and systems have been used by governments, companies, and other institutions for decades by this point; these institutions use AI to boost efficiency through automation. Recently, businesses have started implementing AI in energy management as a way of cutting costs and carbon (Scott, 2019). This article will explore the ways in which organizations implement AI in energy management, namely through the organization of building energy consumption.
The right AI tool will allow organizations to define specific needs of a market, evaluate expertise, consider cost-effectiveness, and access data security, to name a few usages (Scott, 2019). Institutions will often rely on AI for completing tasks that would be time-consuming and not cost-effective. For instance,the emergence of renewables as a commonplace energy source for buildings introduces logistical issues, as it is expensive to provide unlimited energy to institutions, electric vehicles, batteries, and other systems that have a demand for it (Silverstein, 2023). Therefore, distribution systems are required in order to deliver renewable energy in a manner that is economically viable (Silverstein, 2023). These distribution systems require a heavy degree of logistical maintenance, and are typically orchestrated by grid operators, who are tasked in making critical decisions regarding the distribution of energy to recipients. However, as renewable energy distribution becomes increasingly mainstream, it is difficult for grid operators to manage heavily complex channels, making AI an attractive solution.
According to Jeremy Renshaw, senior technical executive for the Electric Power Research Institute, AI technology eases the tasks of grid operators and allows them to make critical decisions in real time (Silverstein, 2023). For instance, AI can gather thousands of pictures of the transmission and distribution system of renewable energy (Silverstein, 2023). Through machine learning, the AI can then create algorithms from these pictures and offer solutions to the operators. This turns out to be a very cost-effective solution for tasks that would otherwise be cost-prohibitive without the presence of AI.
Since the introduction of AI in energy management, complex building energy management systems (BMS) have been introduced. These systems take a step further by catering to a range of consumer behaviour. Different institutions have different building energy consumption patterns. BMSs are capable of delivering energy services to such organizations, exactly when the demand is there (Scott, 2019). One of these systems has been developed by “Flex2X”, developed by Grid Edge, a British company (Scott, 2019). This machine learning system takes data obtained from a building’s existing energy management system and combines it with other data sources. For instance, external conditions such as the weather are used to determine the outflow of energy (Scott, 2019). Flex2X then identifies weather patterns off of these datasets, thus improving energy efficiency. Data suggests that up to 40% of building consumption offsets have been avoided thanks to BMSs (Scott, 2019).
Another important component of BMSs such as Flex2X is the opportunity that it gives for commercial buildings to participate in energy markets (Scott, 2019). When energy consumption is offloaded, managers of commercial buildings can decide to sell their flexible load of energy to the market. This can generate additional revenue streams for sellers, all while reducing energy waste (Silverstein, 2023).
In conclusion, BMSs are an AI tool that have proven to be very useful to companies who put an emphasis on sustainable energy. Such systems prove that AI can be used in a wide variety of situations, and that they can make the lives of humans significantly easier, if used correctly and with the right intentions.
_________________________________________________________________
References:
Scott, Jim (2019). Case Study: Artificial Intelligence for Building Energy Management Systems. International Agency Association. https://www.iea.org/articles/case-study-artificial-intelligence-for-building-energy-management-systems
Silverstein, Ken (2023). How Will Artificial Intelligence Fit Into The Energy Sector? Forbes Business. https://www.forbes.com/sites/kensilverstein/2023/06/07/how-will-artificial-intelligence-fit-into-the-energy-sector/?sh=218c5dfa23cc
Comments